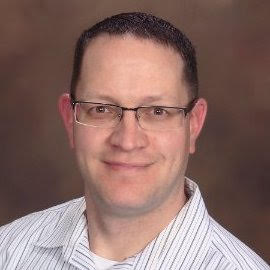
While data is the new currency in today’s digital economy, it’s still a struggle to keep pace with the changes in enterprise data and the growing business demands for information. That’s why companies are liberating data from legacy infrastructures by moving over to the cloud to scale data-driven decision making. This ensures that their precious resource — data — is governed, trusted, managed and accessible.
While businesses can agree that cloud-based technologies are key to ensuring the data management, security, privacy and process compliance across enterprises, there’s still an interesting debate on how to get data processed faster — batch vs. streaming processing.
Each approach has its pros and cons, but your choice of batch or streaming all comes down to your business use case. Let’s dive deep into the debate to see exactly which use cases require the use of batch vs. streaming processing.
What’s the Difference Between Batch and Streaming Processing?
A batch is a collection of data points that have been grouped together within a specific time interval. Another term often used for this is a window of data. Streaming processing deals with continuous data and is key to turning big data into fast data. Both models are valuable and each can be used to address different use cases. And to make it even more confusing you can do windows of batch in streaming often referred to as micro-batches.
While the batch processing model requires a set of data collected over time, streaming processing requires data to be fed into an analytics tool, often in micro-batches, and in real-time. Batch processing is often used when dealing with large volumes of data or data sources from legacy systems, where it’s not feasible to deliver data in streams. Batch data also by definition requires all the data needed for the batch to be loaded to some type of storage, a database or file system to then be processed. At times, IT teams may be idly sitting around and waiting for all the data to be loaded before starting the analysis phase.
Data streams can also be involved in processing large quantities of data, but batch works best when you don’t need real-time analytics. Because stream processing is in charge of processing data in motion and providing analytics results quickly, it generates near-instant results using platforms like Apache Spark and Apache Beam. For example, Talend’s recently announced Talend Data Streams, is a free, Amazon marketplace application, powered by Apache Beam, that simplifies and accelerates ingestion of massive volumes and wide varieties of real-time data.
Is One Better Than the Other?
Whether you are pro-batch or pro-streaming processing, both are better when working together. Although streaming processing is best for use cases where time matters, and batch processing works well when all the data has been collected, it’s not a matter of which one is better than the other — it really depends on your business objective.
However, we’ve seen a big shift in companies trying to take advantage of streaming. A recent survey of more than 16,000 data professionals showed the most common challenges to data science including everything from dirty data to overall access or availability of data. Unfortunately, streaming tends to accentuate those challenges because data is in motion. Before jumping into real-time, it is key to solve those accessibility and quality data issues.
When we talk to organizations about how they collect data and accelerate time-to-innovation, they usually share that they want data in real-time, which prompts us to ask, “What does real-time mean to you?” The business use cases may vary, but real-time depends on how much time to the event creation or data creation relative to the processing time, which could be every hour, every five minutes or every millisecond.
To draw an analogy for why organizations would convert their batch data processes into streaming data processes, let’s take a look at one of my favorite beverages — beer. Imagine you just ordered a flight of beers from your favorite brewery, and they’re ready for drinking. But before you can consume the beers, perhaps you have to score them based on their hop flavor and rate each beer using online reviews. If you know you have to complete this same repetitive process on each beer, it’s going to take quite some time to get from one beer to the next. For a business, the beer translates into your pipeline data. Rather than wait until you have all the data for processing, instead you can process it in micro-batches, in seconds or milliseconds (which means you get to drink your beer flight faster!).
Why Use One Over the Other?
If you don’t have a long history working with streaming processing, you may ask, “Why can’t we just batch like we used to?” You certainly can, but if you have enormous volumes of data, it’s not a matter of when you need to pull data, but when you need to use it.
Companies view real-time data as a game changer, but it can still be a challenge to get there without the proper tools, particularly because businesses need to work with increasing volumes, varieties and types of data from numerous disparate data systems such as social media, web, mobile, sensors, the cloud, etc. At Talend, we’re seeing enterprises typically want to have more agile data processes so they can move from imagination to innovation faster and respond to competitive threats more quickly. For example, data from the sensors on a wind turbine are always-on. So, the stream of data is non-stop and flowing all the time. A typical batch approach to ingest or process this data is obsolete as there is no start or stop of the data. This is a perfect use case where stream processing is the way to go.
The Big Data Debate
It is clear enterprises are shifting priorities toward real-time analytics and data streams to glean actionable information in real time. While outdated tools can’t cope with the speed or scale involved in analyzing data, today’s databases and streaming applications are well equipped to handle today’s business problems.
Here’s the big takeaway from the big data debate: just because you have a hammer doesn’t mean that’s the right tool for the job. Batch and streaming processing are two different models and it’s not a matter of choosing one over the other, it’s about being smart and determining which one is better for your use case.
Feature image via Pixabay.